Measuring translation with ChatGPT 3 AI models to assess quality
Of all the conversations that have been repeated throughout my career in the localization industry, the most frequent (by far) has been about quality—whether localized content is well-localized or not. And I'm not necessarily referring to clear cases of grammatical errors, typos, or things like that, but rather those never-ending discussions related to preferential changes, those comments like, "I don't know, it sounds weird, as if Google Translate did it.
It's true that in the past, there have been models to measure quality, like years ago with LISA or, more recently, with DQF, but the truth is, it's a topic that comes and goes. There have been many attempts to establish localization quality measurement models over the years. Now, during the AI boom, with LLMs in general and ChatGPT in particular, we have a new player entering the scene. So, in the next few paragraphs, I will explore three models where, by using ChatGPT, we can be in a better position to assess whether the quality of our content is up to par or if it's a bit meh...
Let’s explore below three AI-based Language Quality Assessment (LQA) models that are reshaping the landscape of translation quality evaluation.
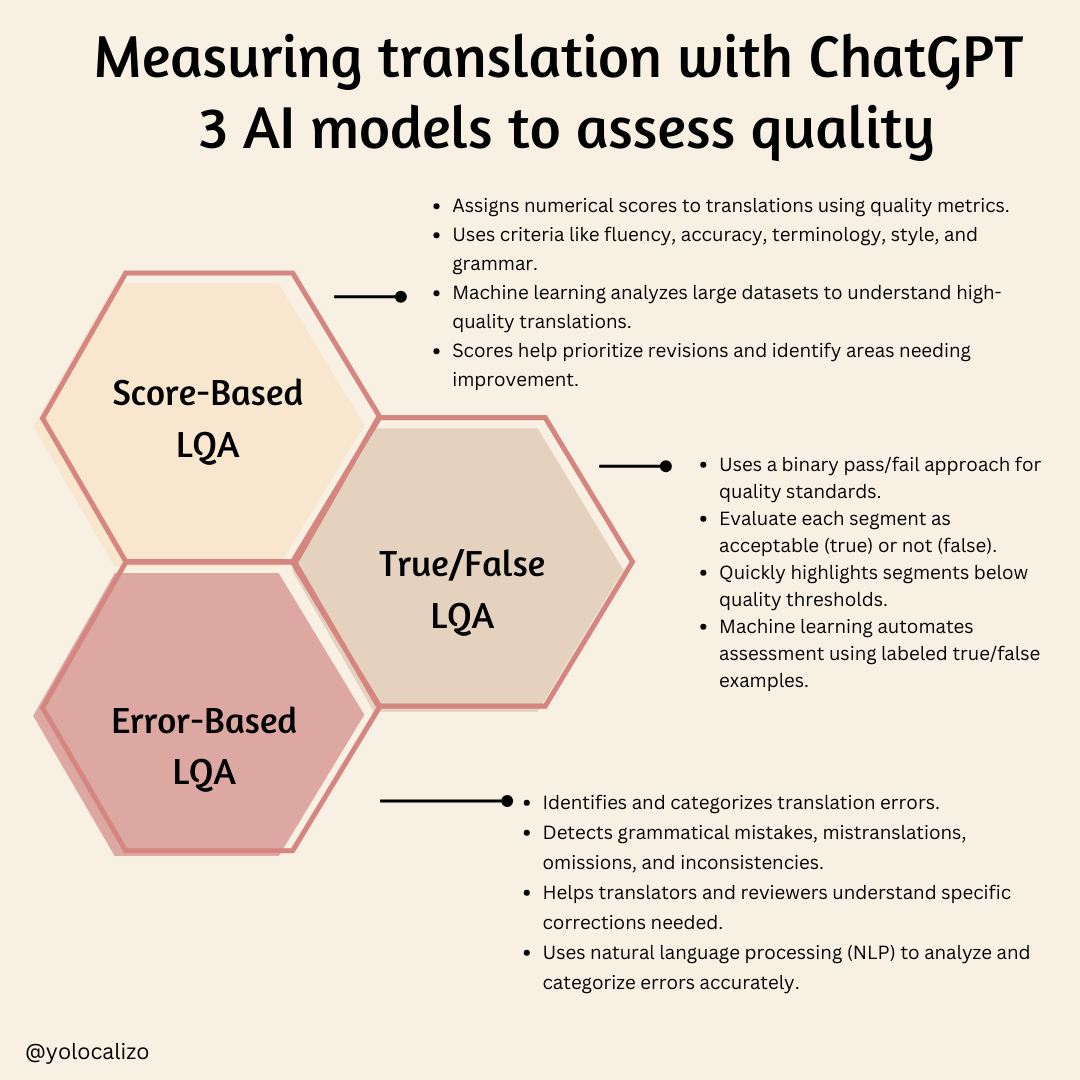




Score-Based LQA
The score-based LQA model assigns a numerical score to translations using a range of quality metrics, such as fluency, accuracy, terminology, style, and grammar. This is a great method to push back “this translation sounds weird” comments.
How It Works
Machine learning algorithms analyze extensive datasets of translated texts to understand what defines high-quality translations. By learning these parameters, the model can score new translations accurately. This scoring helps prioritize revisions and pinpoint areas needing improvement.
Why It Matters
Offers a quantifiable measure of translation quality.
Facilitates systematic improvements in translation processes.
Ensures consistent quality assessments across various projects.
True/False LQA
The true/false LQA model employs a binary approach to determine if translations meet specific quality standards, evaluating each segment as either acceptable (true) or not (false).
How It Works
This method quickly highlights segments that fail to meet the required quality thresholds. Machine learning models trained on datasets with labeled true/false examples automate this assessment.
Why It Matters
Simplifies the quality assessment process with clear pass/fail criteria.
Rapidly identifies problematic segments for immediate attention.
Enhances efficiency by reducing the time needed for manual reviews.
Error-Based LQA
The error-based LQA model focuses on identifying and categorizing translation errors, such as grammatical mistakes, mistranslations, omissions, and inconsistencies.
How It Works
This model pinpoints specific errors, aiding translators and reviewers in understanding exactly what needs correction. NLP techniques are often used to analyze and categorize errors accurately.
Why It Matters
Provides detailed insights into specific translation errors.
Enhances translation quality by addressing precise issues.
Supports translator development through detailed feedback.
SUMMARY
The integration of AI, particularly with tools like GPT and LLMs, marks a major step forward in measuring translation quality. These AI-driven LQA models tackle the long-standing issues in localization quality assessment. Using score-based, true/false, and error-based models can ensure more accurate, efficient, and thorough evaluations of translated content. As these technologies continue to develop, the future of localization looks promising, with AI setting new standards and improving translation quality outcomes.
In this blog post, we explore 3 ideas on how to assess the quality of your localization content with the help of ChatGPT