Fast-tracking our localization career by combining ChatGPT with mental models and cognitive biases
The truth is, watching how ChatGPT evolves is proving to be very interesting, and while it's true that with LLMs we can do interesting tasks in activities related to the most operational aspects of Localization, it's something that doesn't catch my attention as much as experimenting and learning prompts and strategies via ChatGPT that can help me in the area of Localization Strategic Decision Making. I imagine this is because, fundamentally, LLMs are not as revolutionary from an operational perspective as they can be at a strategic level.
Let me explain.
In the localization industry, we've been using machine translation (MT) for decades, and in the case of Neural MT, we've had about a decade or so where the models have advanced significantly to handle many typical translation tasks.
NMT, with its Seq2seq processing model, reminds me somewhat of LLMs. While it's true that the focus of LLMs feels more natural and they resemble a conversation more than Seq2seq models, ultimately, they don't give me a "wow" effect for translating text. NMT was already doing it very well before the chatGPT boom.
But where it does give me a "wow" effect is thinking about how to use AI to help me in areas such as Localization Strategic Decision Making.
The basic principle of learning strategic decision-making is based on understanding the impact they have on the future, the decisions we make today.
But, of course, that's more complex than it seems because of cognitive biases. The topic of cognitive biases is fascinating; we all have cognitive biases, and to make matters worse, we don't know we have them. Identifying them is key since overcoming cognitive biases helps us think more critically and objectively, and we end up making better decisions as a result.
But how can we identify a bias if we don't know we have it?
Until before LLMs, we could achieve this thanks to having people who helped us see what we don't want to see... Or if we are very disciplined by keeping a "decision journal" in which we write down the decisions we make, the results they generate, and whether the result has been optimal or not.
Over time, we will have a “library” of decisions made and a log to analyze the impact.
But now, the truth is that we can make a very powerful combo with chatGPT that allows us to use mental models, and we can also link it to help us analyze and overcome cognitive bias.
Let's see how, with, for example, one of the most popular metal models, the Pareto principle.
The Pareto Principle, also known as the 80/20 Rule, is named after economist Vilfredo Pareto. It asserts an unequal relationship between inputs and outputs, specifically stating that 80% of consequences come from 20% of the causes.
Pareto originally observed this principle in the context of wealth distribution. He found that 80% of the land in Italy was owned by just 20% of the population.
The Pareto Principle serves as a reminder that things in life are not always distributed evenly. It applies to various areas, including manufacturing, management, and human resources.
For instance, in a corporation, 20% of a Product could drive 80% of the firm’s profits.
This can also be applied to personal topics such as time management. Focusing on the most important tasks in our calendar can lead to 80% of work-related output coming from just 20% of your time at work.
Therefore, we can use chatGPT prompts to apply Pareto Principles in our Localization strategy by crafting a prompt to help us with such analysis.
So, a general strategic prompt such as:
"Use the Pareto Principle to find out the small part of [a certain thing or task] that gives most of the results. Then, tell how to focus on that part to get better results."
Can be converted into this prompt applied to Localization
“Apply the 80/20 rule to identify the key components of a localization strategy that yield the most significant impact. Describe how prioritizing efforts on these crucial elements can enhance overall localization effectiveness and efficiency”
Another example is the mental model of the Butterfly Effect, that one not only is it a movie that I really liked in my younger years, but it's also a mental model of strategic thinking that we can use efficiently when explaining certain critical tasks in a localization strategy to illustrate the cause and effect relationship.
Here's another example:
"Use the Butterfly Effect to suggest how small changes in [a situation] could have big effects later."
Adapting that prompt to the world of localization becomes something like...
"Apply the Butterfly Effect to propose how applying Internationalization best practices improvements will lead to significant positive impacts on the overall success of my localization program. Discuss how these seemingly minor changes could ripple out to influence user experience, market penetration, or brand perception in target regions."
And the result we receive gives us good food for thought.
There are many mental models; I usually guide myself with the recently deceased :( Charlie Munger, the inseparable companion of Warren Buffet (who is said to be the best investor who ever existed), who encourages us in his books to learn mental models and to use those that are most useful to us by testing them and keeping those that are most useful.
Source: sloww.co
Cognitive biases – the danger of what we won’t see
Regarding cognitive biases, the idea is exactly the same; we must seek the biases we want to explore and then adapt them. For example, the model I most fall into is confirmation bias.
Confirmation bias is a common cognitive tendency where individuals seek, interpret, and favor information that aligns with their existing beliefs or values.
An example of my confirmation bias in the past has been related to confirmation bias in Localization automation solutions.
In the past, screenshot automation for LQA worked for me; therefore, I always assumed that implementing automation solutions would inherently improve efficiency and quality without thinking about the time needed to create scripts to capture every dialog I wanted to be tested.
A great list of cognitive biases can be found in this infographic that I attach below.
Source:BrillanceBrief.com
Conclusion
Learning to use ChatGPT alongside mental models and cognitive biases can significantly enhance our ability to develop a successful localization strategy program. By understanding cognitive biases, we can recognize and address potential pitfalls in decision-making, ensuring our localization efforts align with strategic objectives.
Let’s be creative and witht the help of 🤖find ways to become better strategists!
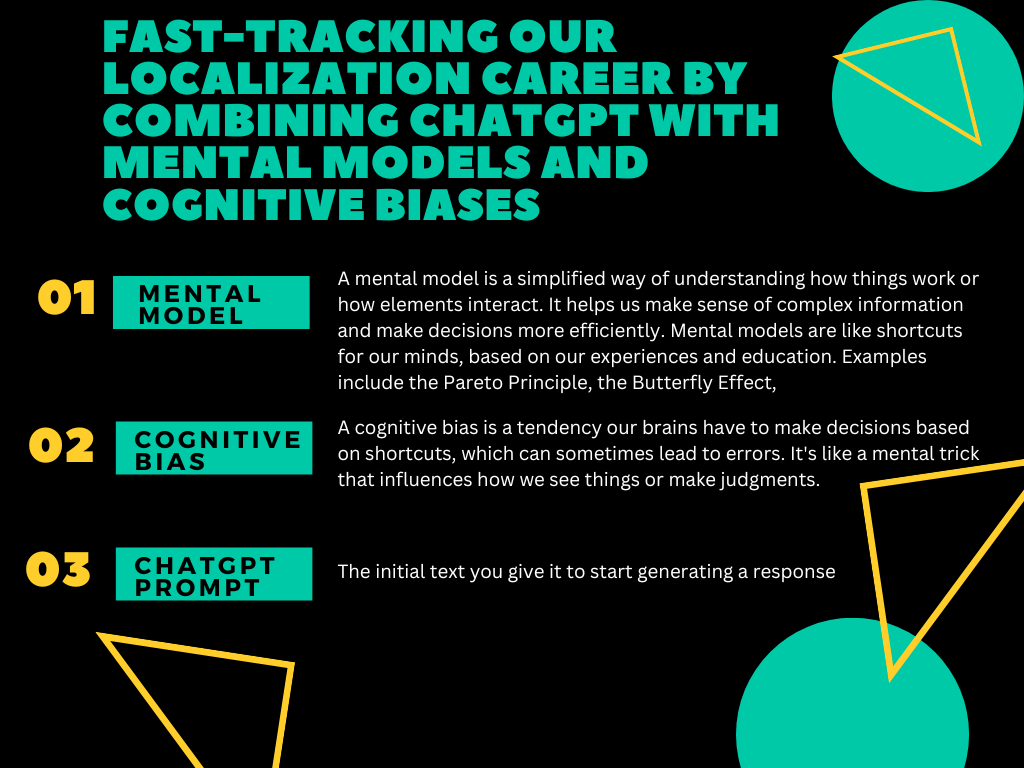
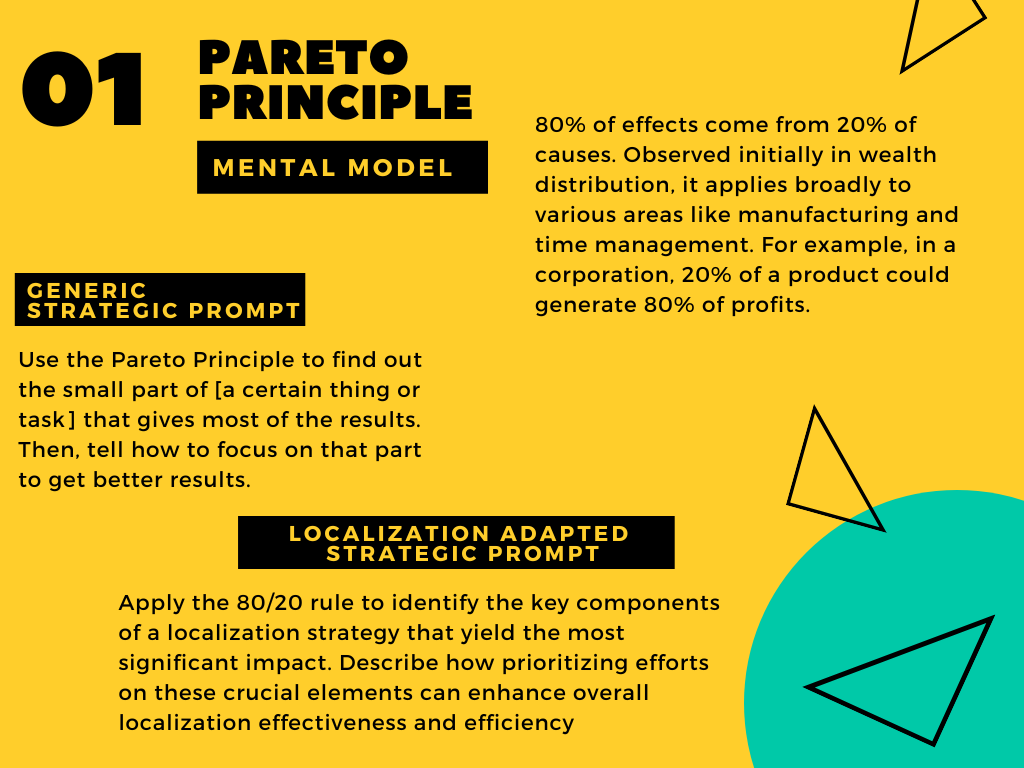
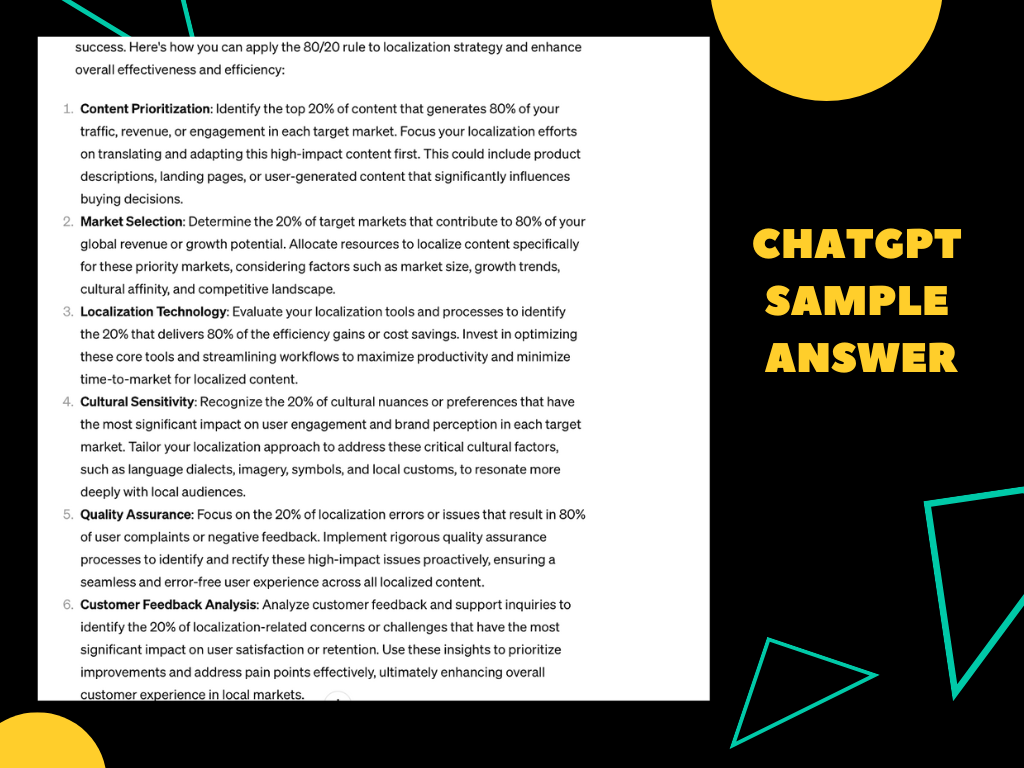
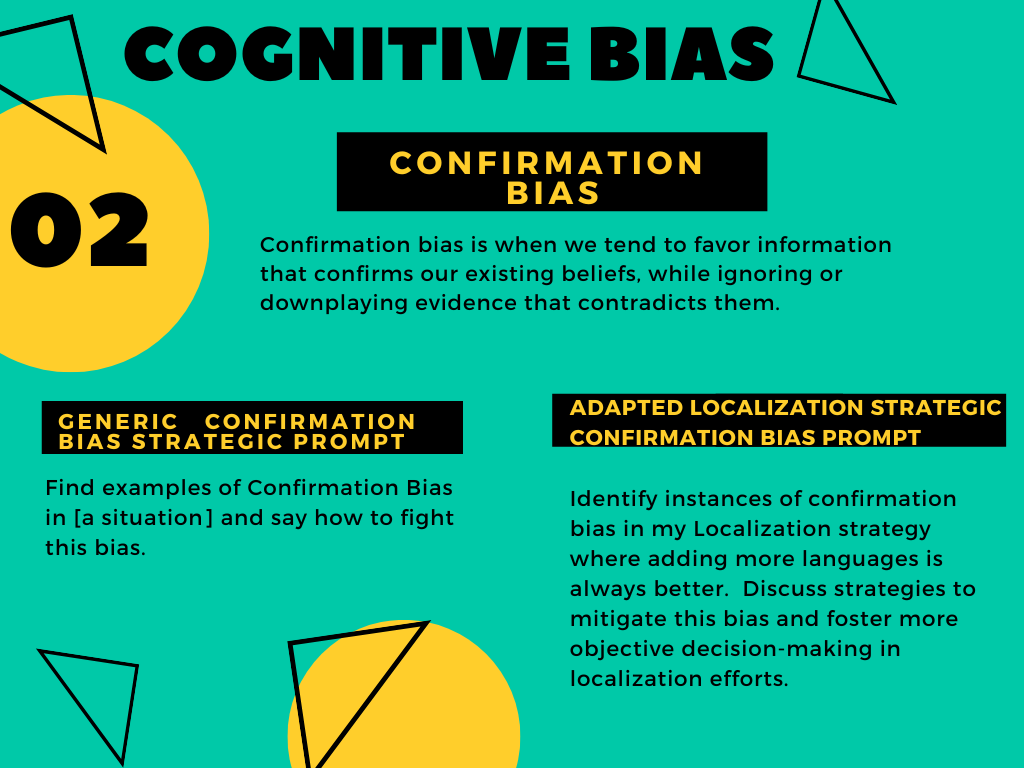
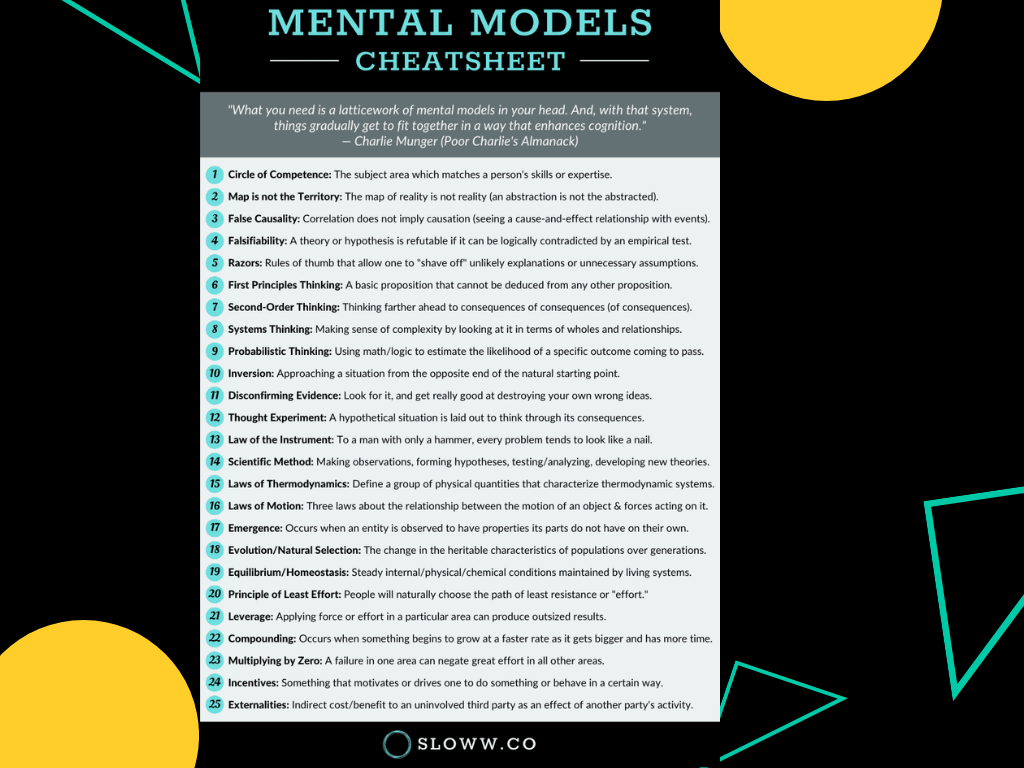
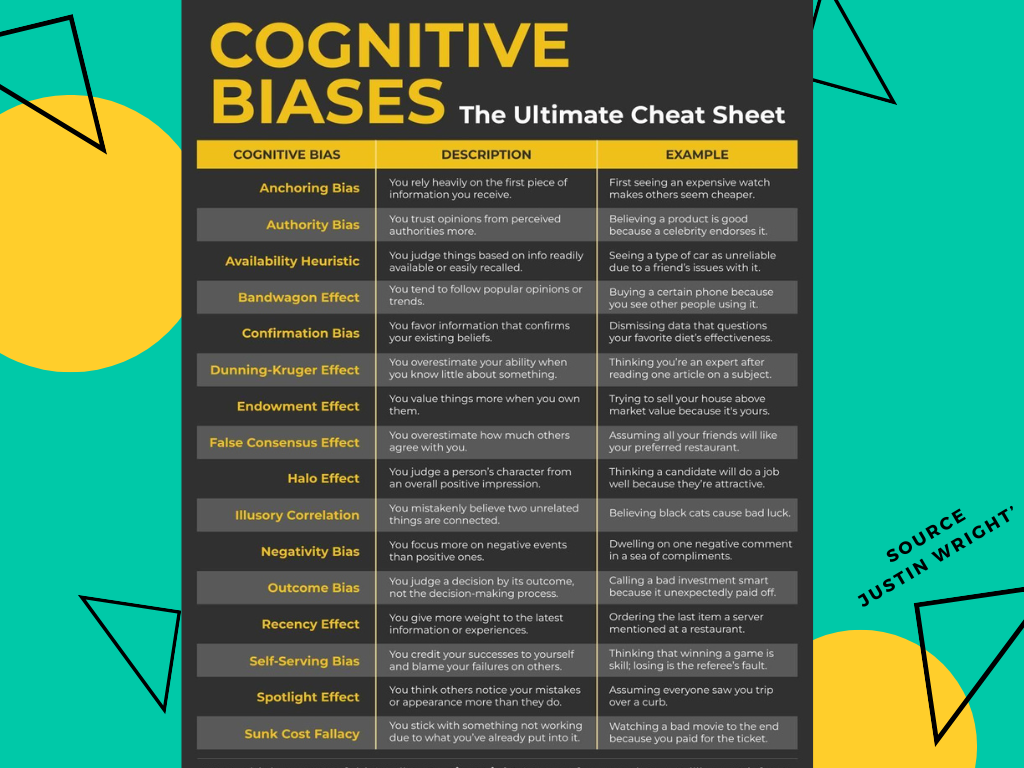
If we want to treat localization like a product, we need to measure it like one. Traditional metrics like cost per word and delivery time still matter, but they don’t capture the full picture. In this post, I share four categories of product-style metrics: adoption, automation impact, strategic influence, and internal visibility that they can help you track the real value your localization team is creating across the company.